Kate Crawford | Trevor Paglen
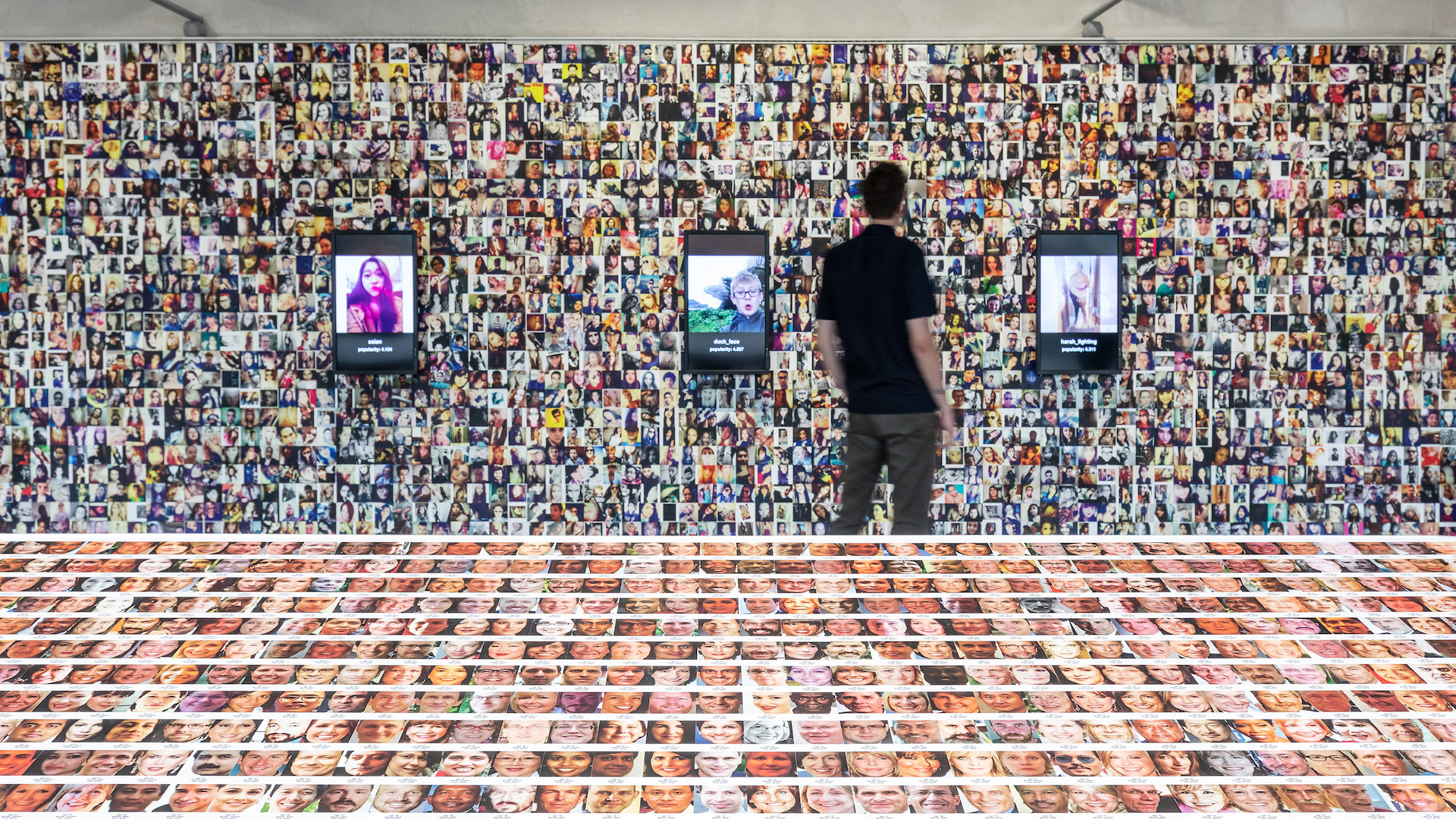
The Image-Force
So here’s the situation. We are in a place named Osservatorio (which is not a former observatory), looking at pictures not intended to be looked at as art, but displayed as such, though they do not claim to be art, and the artists/authors of the exhibition don’t claim they are either. Most of the pictures in this exhibition are portraits, with the exception of some historical documents.
The first series of faces we come across has been extracted from the FERET dataset, a collection of 14,126 facial images of 1,199 individuals gathered between 1993 and 1996. The FERET program—which stands for Face Recognition Technology—was a US Department of Defense research program aimed at developing “automatic face recognition capabilities that could be employed to assist security, intelligence, and law enforcement personnel in the performance of their duties”1. It gave rise to the first “large” database created with the general purpose of improving facial recognition compared to the usual datasets created by developers to train and test the precise algorithms they were programming. For the National Institute of Standards and Technology, “Before the start of the FERET program, there was no way to accurately evaluate or compare facial recognition algorithms.”2 And that’s precisely what is at stake in this exhibition by multidisciplinary AI researcher and professor Kate Crawford and artist and researcher Trevor Paglen: the question of objectivity in facial recognition algorithms.
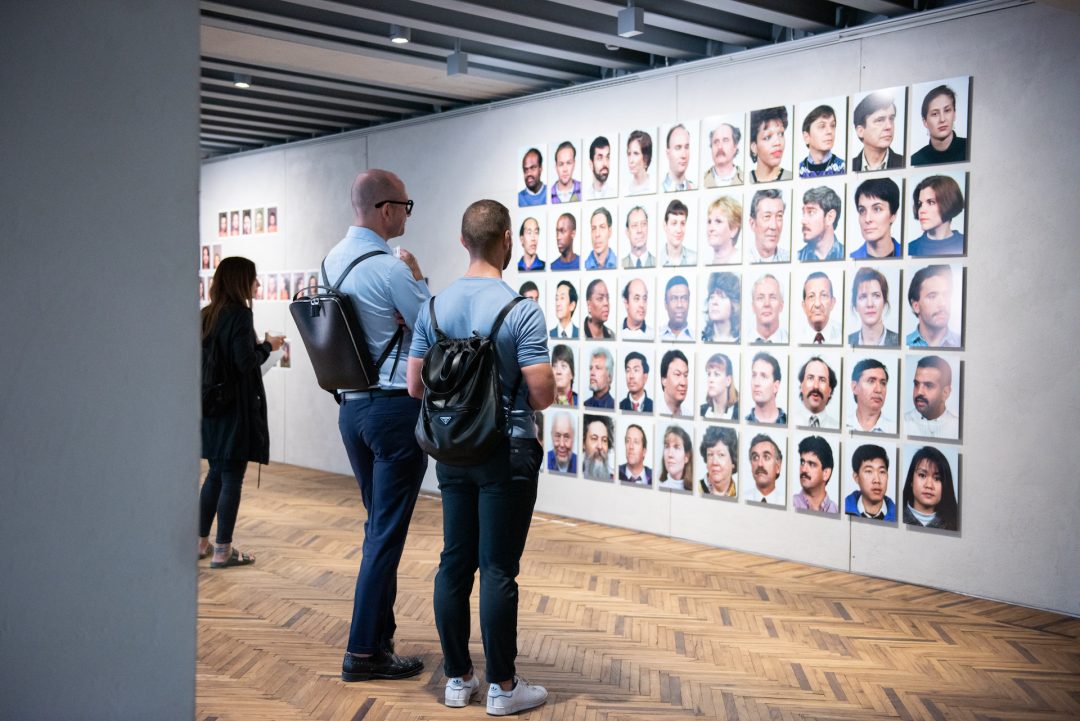
The second excerpt of a dataset on view is more straightforward in this regard: it consists of pictures of deceased persons arrested multiple times, which allow for the tracking of changes in their faces, such as hair growth or aging. The MEDS-II (for Multiple Encounters Dataset-II) was created in 2011 using images and metadata provided by the FBI, i.e. images not produced on purpose but recycled—or upcycled, depending on your personal views. As this dataset was also assembled in the US, it features mostly white and black men, very few women and even less Asian people. As the social networks and image hosting websites were still in their infancy (Facebook and Flickr were only five years old when the first MEDS files were gathered), where could one look for pictures of the same persons over a significant period of time with attached data? There were two main options: celebrities and convicts.
It may have been easier for the government to opt for the second solution as the database was in some way already existing and verified. Which doesn’t mean it was fairly representative. As Trevor Paglen humorously points out: “Those two datasets are maintained by the National Institute of Standards of the US, they are to facial recognition what the kilogram is to mass!”3
The creation of standards for machines is obviously a crucial point. So how can things be reduced to data gathered from a few hundred people working in a university or in the military willing to donate the image of their face to advance research?
The celebrities option was actually chosen by four researchers who, in 2007, published a dataset titled Labeled Faces in the Wild, a collection of 13,233 images of 5,749 celebrities “spanning the range of conditions typically encountered in everyday life” as opposed to “most face databases [that] have been created under controlled conditions to facilitate the study of specific parameters on the face recognition problem.”4 Crawford and Paglen display its pictures next to the Selfie dataset which, as its name implies, is a database of selfies. But were those 46,836 selfies donated to advance research? Well, no. They were scraped from Instagram by the Center for Research in Computer Vision at the University of Central Florida.
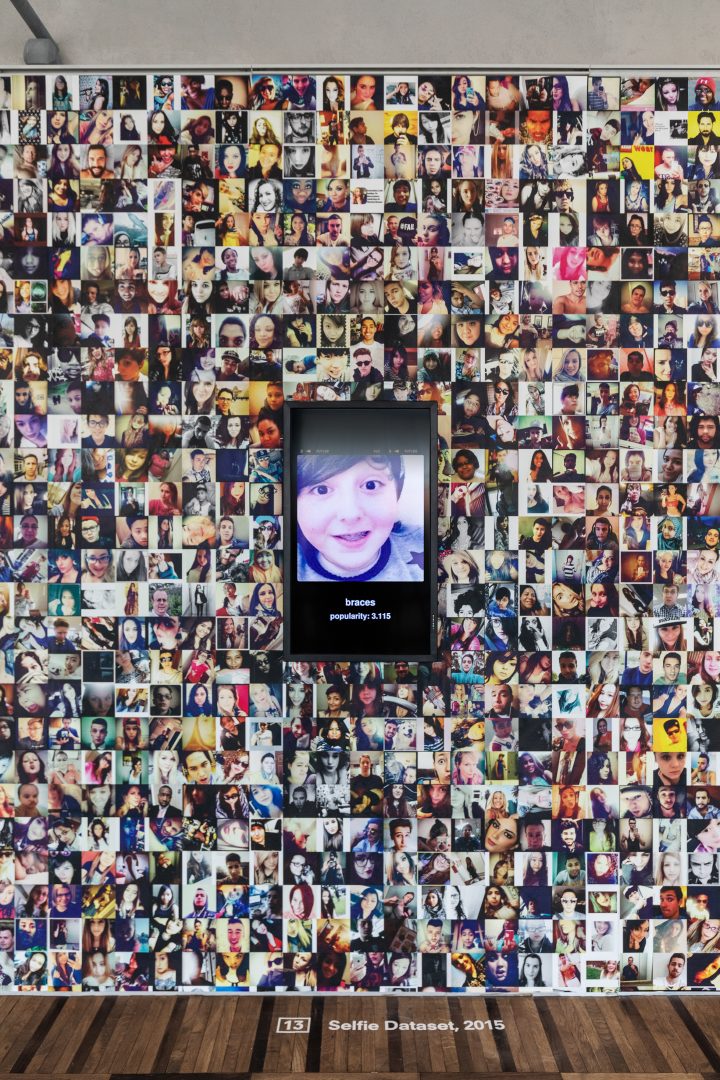
2015 did indeed mark the release of two datasets of portraits of living “anonymous” persons whose pictures were gathered without their consent: the Selfie dataset and the Brainwash dataset, this latter being composed of pictures extracted from a webcam broadcast from a San Francisco café. While the Brainwash dataset, produced by Stanford University, was removed from its original website in June 2019 after being exposed by artists and researchers Adam Harvey and Jules Laplace5 as notably used by the National University of Defense Technology in China and by researchers affiliated to Megvii, a Chinese leading AI company that provides facial recognition to Lenovo and Huawei among others, the Selfie dataset is still available. It should be noted that we are here only mentioning publicly available research datasets but, of course, there are dozens of proprietary datasets whose existence we more or less ignore, such as the ones used internally at Google or Facebook, for instance.
Let’s not forget that “Facebook has the biggest collection of human faces in all history!” indicates Kate Crawford, “an archive of so many human faces that is privately held, that it can possibly compares to the gigantic collections of the most powerful European families in history: there’re like the Medici of faces!”6 she wittily adds.
Displaying excerpts of such data sets in an art space raises of course classical art world questions: What is the difference between looking at a photograph that we know is art and looking at a random selfie of someone we don’t know on a social network?
But, here, the situation raises more urgent questions as we are thus looking at pictures that are mostly looked at by machinic eyes, that may have been produced by humans for humans but that end up being used by machines to look at humans.
A few years ago, in an insightful essay, Trevor Paglen warned that: “Human visual culture has become a special case of vision, an exception to the rule. The overwhelming majority of images are now made by machines for other machines, with humans rarely in the loop.”7 Would that mean that images are becoming more and more operative? The aim of the images thus named by Harun Farocki8 at the dawn of the millennium, is not representation but action, active participation in human affairs. This idea was not exactly new, in fact, it is even already sixty years old. It’s now sixty years since images began to elude us.
At the origin of this revolution, among a few scientific and technological breakthroughs in the domain, was a study by neurobiologists David H. Hubel and Torsten Wiesel, published in 1959, on the processing of information by neurons in the cat’s visual cortex9. Their discovery of the impact of the visual environment on the structuring of vision and its functioning by analyzing layers of information, combined with the results of neurologists’ research on the neural networks of frogs10, also unveiled in 1959, formed the basis of what is known as deep learning in artificial intelligence. Deep learning algorithms, present in the majority of artificial neural networks (also actually implemented in 1959)11, are used in many fields, from automatic translation to personal assistants by way of trading, to quote only a few, but here we will focus on computer vision. Just as the functioning of artificial neural networks is based on the (supposed) functioning of natural neurons, computer vision is inspired by the refinement of visual information in the human12 and animal13 cortex. For this purpose, the image run into the system, like the perceived image, is broken down into different elements: in a nutshell, shapes, relief and colours. Of course, in order to be understandable by the machine, it is translated into sequences of numbers, equations and other functions, a process developed in 1957 by engineer Russell A. Kirsch, creator of the digital scanner and thus of the first digital image. “What would happen if computers could see the world as we see it?”14 It was to answer this question that he set out to divide his son’s now-famous photo into small squares with mathematically describable properties: pixels.
Around the same time, a mathematician and computer scientist at work on machine reading and more particularly on character recognition, was being ambitious ; about those research years and the dreams he had, Woodroe W. Bledsoe wrote: “I wanted it to read printed characters on a page and handwritten script as well. I could see it, or a part of it, in a small camera that would fit on my glasses, with an attached earplug that would whisper into my ear the names of my friends and acquaintances as I met them on the street… For you see, my computer friend had the ability to recognize faces.”15 So in 1960, together with two of his colleagues at the national nuclear security research and development laboratory he was working at, he incorporated Panoramic Research in Palo Alto—right at the start of the silicon revolution16, though before the Valley was thus named—, a company dedicated to speculative research in artificial intelligence and robotics, if we have to summarize things. Three years later, Bledsoe published “A Facial Recognition Project Report”, which is still today the first known attempt to devise “a solution of a simplified face recognition problem”17. Apart from describing a way to transpose the pattern recognition method created by Bledsoe, for a computer to recognize letters, to images of human faces, this document also highlights the necessary human work for the functioning of the system: “It is felt that for an eventual recognition machine a certain amount of work by humans may be highly profitable. For example, when giving the machine a picture to be identified, the machine operator might be asked to give the sex (if known), race, etc. and to provide scaling information.”18
This facial recognition project as well as a large part of the work conducted by Panoramic Research has been funded by King-Hurley Research group, an alleged front company for the CIA19, which should be the reason why very few research papers by Bledsoe have been published so far20. One of them, the aforementioned “Facial Recognition Project Report”, opens “Training Humans”, the Paglen-Crawford exhibition at Osservatorio, which made Trevor Paglen say, in an acerbic tone: “When you’re looking at the very foundations of artificial intelligence and computer vision research, you find the CIA and some very cruel experiments on cats, that’s the ground upon which all of it is built.”21 Thus it might not be that much of a surprise to read the name of Alphonse Bertillon in the bibliography of this report, 22 any more than, maybe, to discover that Panoramic has apparently also conducted research in the framework of the infamous MK-Ultra project, the “mind control-brainwashing” CIA research project that led to the formalization of interrogation techniques still in use23.
If Belgian mathematician Adolphe Quételet was the first to apply statistics and probabilities to psychology but also to criminology, by way of measurements of the human face and body among other questionable techniques—funnily enough, he also created the BMI— systematizing the use of the body in the identification process of a person, he largely influenced Bertillon, the French creator of judicial anthropometry, a classification system widely adopted by police departments from the 1890s. We can obviously read the biometric information embedded in the chips in our passports as a contemporary version of the Bertillon system. Measuring distances between one eye and another, between the nose and the upper lip and using this data to identify people, was not only used in the nineteenth century to convict repeat offenders or by Bledsoe to teach his computer friend to recognize faces, it is still at the core of today’s facial expression recognition systems.
Not only are emotion detection algorithms mainly used “against” the people they’re analyzing—to find out whether people are being honest in their reponses during a job interview24 or to better target them as a customer, among many other examples—but they are absolutely flawed. Take for instance the JAFFE (for Japanese Female Facial Expression) database which compiles 213 pictures of psychology students at Kyushu University labelled with “the predominant expression […] that the subject was asked to pose”, the six emotions expressed being: happiness, sadness, surprise, anger, disgust and fear. Although this database was released in 1998, the exact same categories and the exact same five levels of appreciation it uses are currently used by human-in-the-loop clickwork companies for emotion detection. The question is: what allows one to infer somebody’s inner state from their facial expression?
Grounding a multibillion-dollar market onto “six universal emotions” sounds at the very least preposterous. Next to the JAFFE database, Crawford and Paglen have chosen to exhibit the UTKFace database categories in order to underline the “profound simplification of human complexity to make it readable by AI”25 to use Crawford’s words. UTKFaces classifies people into two genders and five races: white, black, Asian, Indian, and other. To go on quoting Crawford: “AI systems reencode these types of very narrow understandings of humanity”.
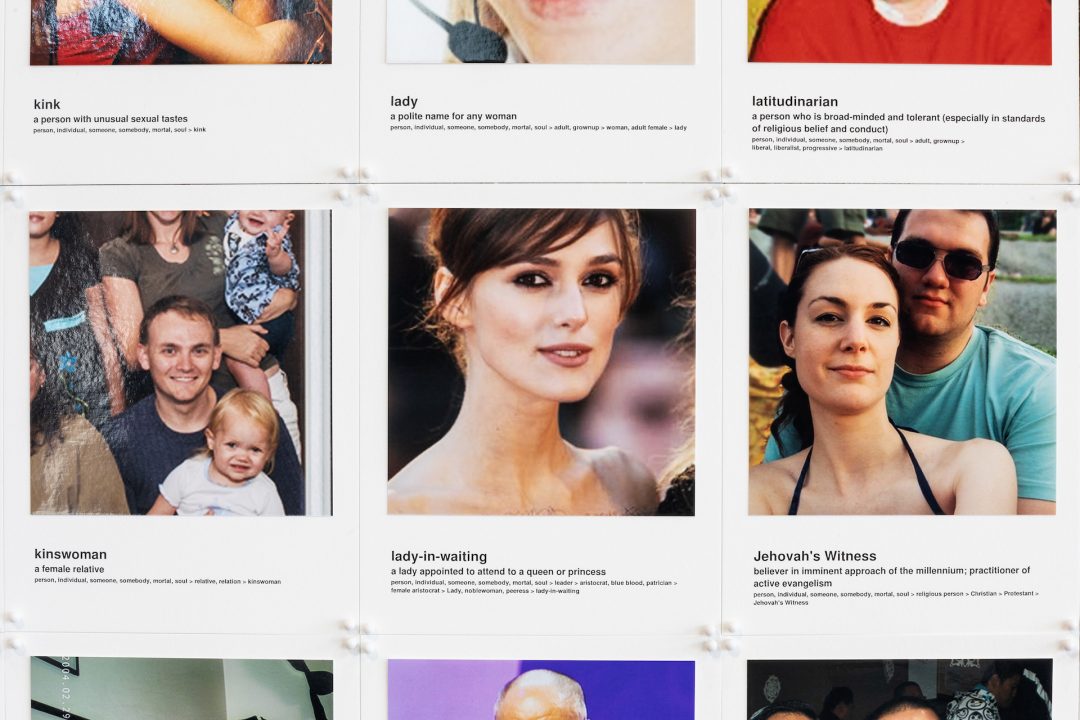
But classification goes further, as Paglen and she demonstrate by describing the most famous of the datasets on view in “Training Humans” and probably the most famous dataset, period: ImageNet. The idea behind this gigantic dataset created in 2009 at Stanford Univesity was “to catalog the entire world of objects, to get images of every possible object in the world in order to be able to identify it: to this day, there are 14,1 million images categorized in about 20 000 categories, the majority of those being fruits, animals, ustensils… And more than 2800 categories are kinds of people: men, women, trumpeter, debtor—somebody who is indebeted, but how would you know what is going on on somebody’s bank accound by looking at their face?”26asks Trevor Paglen.
More precisely, ImageNet is an image dataset organized according to the WordNet hierarchy, a database containing most everyday words in the English language. Each meaningful concept in WordNet, possibly described by multiple words or word phrases, is called a ‘synonym set’ or ‘synset’. There are more than 100,000 synsets in WordNet, and the aim of ImageNet is to provide on average 1,000 images to illustrate each synset. To do so, ImageNet compiles an accurate list of web images for each of them. “Images of each concept are quality-controlled and human-annotated”27 stresses its website, emphasizing, if it were still necessary, that there is no real artificial intelligence but only man-machine systems. Which doesn’t make those systems smarter, it has to be said. As, “for example, alongside categories like cheerleader, or doctor, or politician, there are also categories for alcoholic, for bad person, for kleptomaniac, for fascist, for liar, for loser… You’ll see errors of language, errors of representation, many categories that are completely non visual. Even a picture of a person who’s called colorblind. What does a colorblind person look like?” explains Kate Crawford. “What you see with ImageNet is an extreme example of what is going on in all of the training sets in general which is that, at some point, a description of a person transforms into a judgement over them”28 adds Paglen.
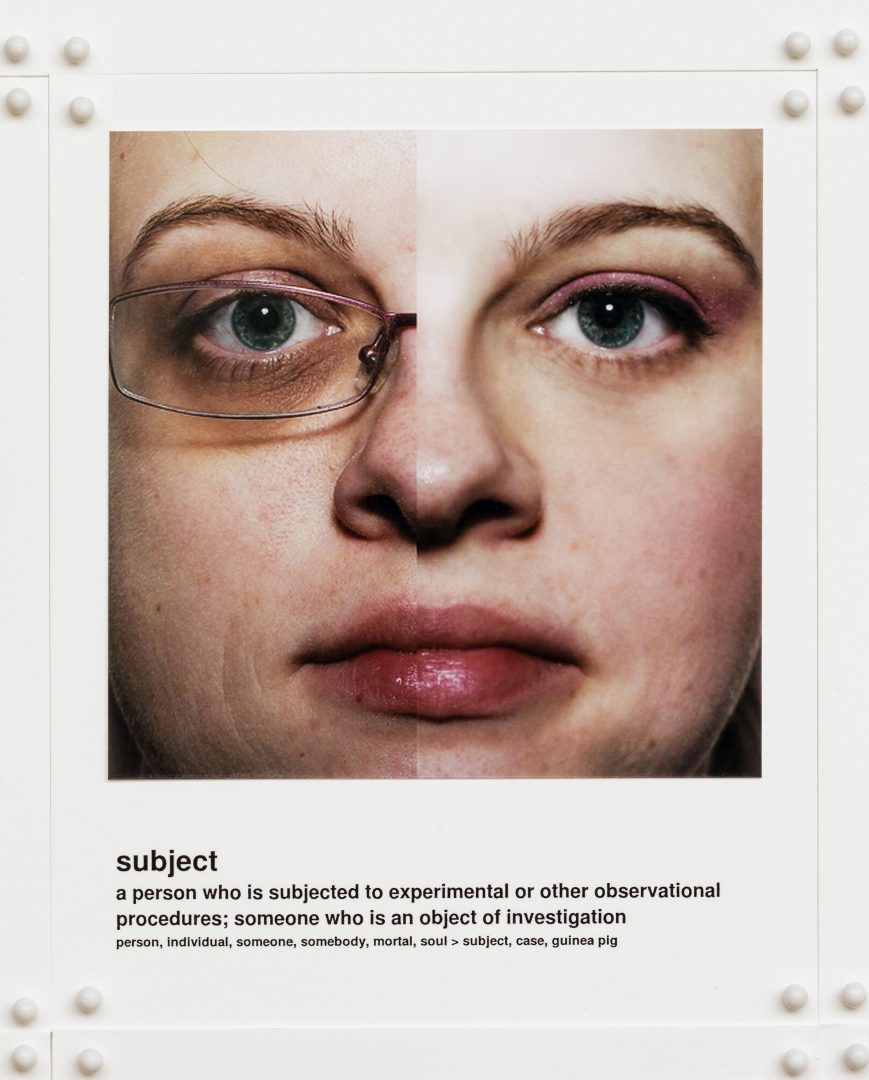
Standing in front of a house makes you an inhabitant, close to a child makes you a loved one, close to a knife makes you a murderer, close to a phone makes you a call girl. Holding a microphone makes you an announcer, holding a snake makes you a charmer. This is all very literal. But wearing glasses and looking Asian makes you an abstractor. You asked for clichés? Kate Crawford, who I already quoted in these pages on this very same topic a few years ago29 has long been struggling for the biases in AI to be acknowledged but, as she recalls, “when I first said it, it was a heresy”30.Which is what led her to co-create the AI Now Institute, “an interdisciplinary space so that sociologists, anthropologists, philosophers and artists—we also invited Trevor to join us—could be part of that institute that would create critical AI studies”31.
To make those ideas more graspable, Trevor Paglen and his studio team created Image-Net Roulette, a face detectionneural network trained on the persons categories from ImageNet. You face its camera and its camera detects your face and compares it to the faces with which its model has been trained. Then it shows you the categories it guesses you belong to.
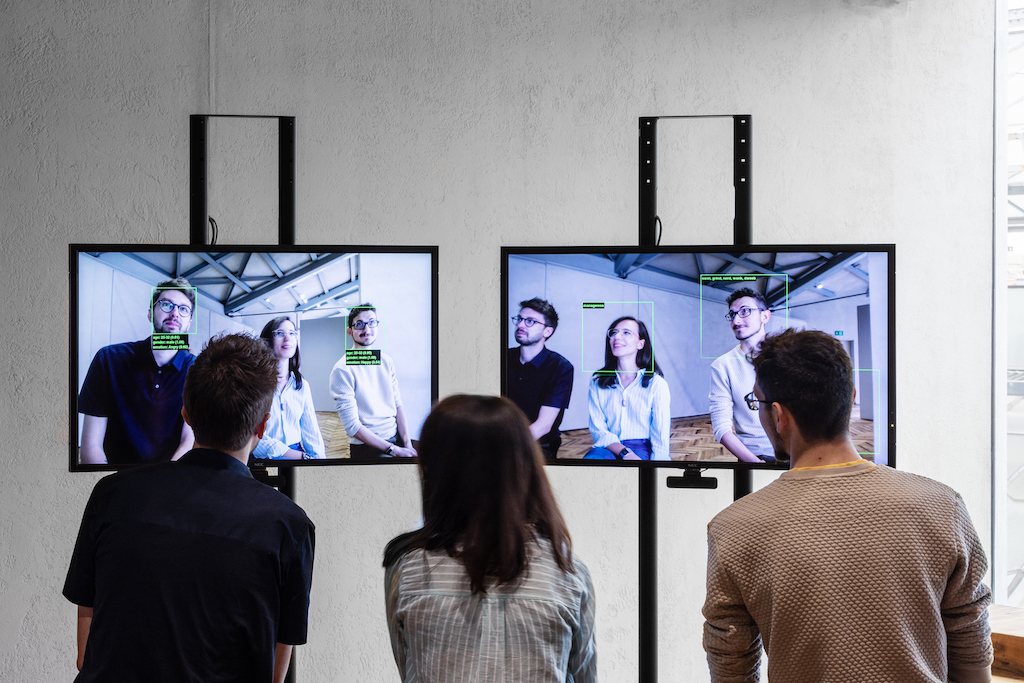
I have personally been recognized by the system as a psycholinguist, a nonsmoker, a co-pilot, a flatmate, a schizophrenic, a divorced man, a grass widower, a grammarian, a flight attendant, a passenger rider, an extrovert, a redneck cracker, a soul mate, a big sister, a little brother, a namby-pamby, a hypnotizer, a common person, a pessimist, a call girl (when I was holding my phone in an obvious way), a sleeper, a slumberer, a utilizer, and, if I started smiling, the system saw me as a careerist and a sociologist. There was a woman in front of me, she had a big forehead but long and thick hair, and she was labelled a skinhead. When I tell Paglen and Crawford about it, this latter blurts out: “I got chess master yesterday!” and, turning to Paglen: “You got white supremacist” which he can only confirm with a droll
“I did.”
“The vast majority of the models in facial recognition are standardized, so most of computer vision, even done by companies, is based on the same models” he goes on explaining.
And, as I was wondering how many people actually have access to the proprietary ones that are used internally at Google or Facebook, Kate Crawford responded: “If you aggregate the technical workforce of the Big Five, you won’t even get a hundred thousand. And that’s the number of people who are creating systems of visuality that are influencing the lives of billions of people.”
Six decades after the first experiments in computer vision, the question is now: how did we make machines see us? As operative images are mainly invisible, never brought to our human eyes as they don’t even need to take the form of what we call images to complete the mission they are collected for, we can only wonder, together with Trevor Paglen: “if AI is trained on images like these and then put out into the world and used, how is AI actually training us?”
In the wake of the news of the EU considering the creation of a “network of national police facial recognition databases”32, this reflection has unfortunately only just begun.
As I pass by Image-Net Roulette one hour later, the system recognizes me: “grammarian, nonsmoker, psycholinguist”. It also frames an empty part of the wall behind me and labels it “painter”.

1 As presented on the National Institute of Standards and Technology website, https://www.nist.gov/programs-projects/face-recognition-technology-feret
2 Idem.
3 Trevor Paglen during the presentation of the exhibition.
4 Gary B. Huang, Tamara Berg, Marwan Mattar, Eric Learned-Miller, “Labeled Faces in the Wild: A Database for Studying Face Recognition in Unconstrained Environments”, October 2008.
5 https://megapixels.cc/brainwash/
6 Kate Crawford, interview by the author, Milan, Sept 11, 2019.
7 Trevor Paglen, “Invisible Images (Your Pictures Are Looking at You)”, The New Inquiry, December 8, 2016, https://thenewinquiry.com/invisible-images-your-pictures-are-looking-at-you/
8 “In my first work on this subject, Eye/Machine (2001), I called such pictures, made neither to entertain nor to inform, ‘operative images.’ These are images that do not represent an object, but rather are part of an operation.” Harun Farocki, “Phantom Images”, Public, n° 29, New Localities, 2004, p. 17.
9 David Hubel and Torsten Wiesel, “Receptive fields of single neurones in the cat’s striate cortex”, The Journal of Physiology, 1959, n°148, p. 574-591. https://www.ncbi.nlm.nih.gov/pmc/articles/PMC2718232/
In the exhibition Training Humans, Trevor Paglen and Kate Crawford present a video from 1973 of—rather cruel—experiments conducted on kittens by Colin Blakemore, a neurobiologist who was studying development in the visaul cortex. https://www.youtube.com/watch?v=QzkMo45pcUo
10 Jerome Lettvin, Humberto Maturana, Warren McCullloch and Walter Pitts, “What the frog’s eye tells the frog’s brain”,1959,Proceedings of the Institute of Radic Engineers, vol. 47: 1940-1959. https://neuromajor.ucr.edu/courses/WhatTheFrogsEyeTellsTheFrogsBrain.pdf
11 MADALINE (for Multiple ADAptive LINear Elements) was the first artificial neural network applied to a real-world problem. Developed in 1959 by Bernard Widrow and Marcian Hoff at Stanford, its architecture is still in use today.
12 “The retina sends very little information to the brain; what we see is actually reconstructed by our brain”, as Gérard Berry explains in La photographie numérique, un parfait exemple de la puissance de l’informatique (Digital photography, a perfect example of the power of computers), his lecture at the Collège de France on January 31, 2018. https://www.college-de-france.fr/site/gerard-berry/course-2018-01-31-16h00.htm
13 Some assertions by Lettvin, Maturana, McCullloch and Pitts have since been contradicted, see: Conor Myhrvold, “In a Frog’s Eye”, MIT Technology Review, January 2, 2013.https://www.technologyreview.com/s/508376/in-a-frogs-eye/
14 Russell A. Kirsch quoted in Rachel Ehrenberg, “Square Pixel Inventor Tries to Smooth Things Out”, Wired, June 28, 2010. https://www.wired.com/2010/06/smoothing-square-pixels/
15 Woody Bledsoe, in an unpublished essay from 1976, quoted in Shaun Raviv, “The Secret History of Facial Recognition”, Wired, January 21, 2020. https://www.wired.com/story/secret-history-facial-recognition/
16 The metal-oxide-silicon field-effect transistor—the first miniature transistor— was indeed conceived in 1959 and led to the massive development of electronic devices that we know.
17 Woodroe Wilson Bledsoe, “A Facial Recognition Project Report”, 1963, p. 1.https://archive.org/details/firstfacialrecognitionresearch/mode/2up
18 Idem, p. 2.
19 Bill Richards, Firm’s Suits Against CIA Shed Light on Clandestine Air Force, The Washington Post, May 12, 1978. https://www.washingtonpost.com/archive/politics/1978/05/12/firms-suits-against-cia-shed-light-on-clandestine-air-force/a8d77856-6920-40a4-a430-13b9c9171245/
The information has been neither confirmed nor denied by the CIA: https://www.muckrock.com/foi/united-states-of-america-10/relationship-of-king-hurley-research-group-and-cia-10656/
20 Woody Bledsoe’s son has reported having seen his father set fire to a certain amount of documents, some of which marked ‘Classified’, in 1995; see Shaun Raviv, “The Secret History of Facial Recognition”, art.cit. The rest of his archive is held at the Briscoe Center for American History at the University of Texas in Austin.
21 Trevor Paglen during the presentation of the exhibition.
22 Woodroe Wilson Bledsoe, “A Facial Recognition Project Report”, 1963, Bibliography, p . I.
23 See for instance the now declassified (since January 1997) Kubark Counterintelligence Interrogation manual, dated July 1963. https://nsarchive2.gwu.edu//NSAEBB/NSAEBB122/index.htm#hre
24 Patricia Nilsson, “How AI helps recruiters track jobseekers’ emotions”, Financial Times, February 28, 2018.https://www.ft.com/content/e2e85644-05be-11e8-9650-9c0ad2d7c5b5
25 Kate Crawfordduring the presentation of the exhibition.
On this precise question, see L.F. Barrett, R. Adolphs, S. Marsella, A.M. Martinez, and S.D. Pollak, “Emotional expressions reconsidered: Challenges to inferring emotion from human facial movements”, Psychological Science in the Public Interest, 20, 2019, p. 1-68.
26 Trevor Paglen during the presentation of the exhibition.
27 http://image-net.org/about-overview
28 Bothduring the presentation of the exhibition.
29 Aude Launay, “Algocurating”, 02 n°80, Winter 2016-17.
30 Kate Crawford, interview by the author, Milan, Sept 11, 2019.
31 Idem.
NB All the following quotes are excerpts of the conversation that followed my interview with Trevor Paglen and Kate Crawford in Milan.
32 Zach Campbell, Chris Jones, “Leaked Reports Show EU Police Are Planning a Pan-European Network of Facial Recognition Databases”, The Intercept, February 21, 2020. https://theintercept.com/2020/02/21/eu-facial-recognition-database/
Kate Crawford | Trevor Paglen, Training Humans, Fondazione Prada Osservatorio, Milan, 12.9.2019 – 24.2.2020
Panel discussion with Kate Crawford and Trevor Paglen moderated by Aude Launay on Wednesday April 8, 2020, 7 pm, Jeu de Paume Auditorium, Paris >POSTPONED, date TBA.
Image on top: On the table : UTKFaces, Zhifei Zhang, Yang Song, Hairong Qi, 2017. On the wall : Selfie Dataset, Mahdi M Kalayeh, Misrak Seifu, Wesna LaLanne, and Mubarak Shah, 2015. Exhibition view of Kate Crawford | Trevor Paglen: Training Humans, Osservatorio Fondazione Prada. Photo : Marco Cappelletti. Courtesy Fondazione Prada.
- From the issue: 93
- Share: ,
- By the same author: Thomas Bellinck, Christopher Kulendran Thomas, Giorgio Griffa, Hedwig Houben, Pamela Rosenkranz,
Related articles
Céleste Richard Zimmermann
by Philippe Szechter
Julien Creuzet
by Andréanne Béguin
Anne Le Troter
by Camille Velluet